BFP #OpenLP Series (10) – Our advice when it comes to Quantitative Approaches in general is to focus on building a compelling narrative around your unique differentiation rather than around an AI model which everyone else can replicate.
Venture Capital in the Era of Big Data
In the era of big data and AI, not surprisingly we started to see more and more venture capital managers adopting new quantitative investment processes and frameworks.
As of today, we are intrigued but not nearly convinced by what we have seen so far.
Certainly we consider quantitative frameworks as useful supplement of a good investment process, but as a Limited Partner we strongly recommend to “advertise” these frameworks as a support tool rather than a major competitive advantage.
The data models we find in the market are substantially of two types:
1. Scouting and filtering models
2. Quantitative approaches to decision making for the investment (i.e. when deciding about a deal)
This article (Part 1) will briefly cover the scouting and filtering models, while Part 2 will touch upon quantitative approaches to investment decisions.
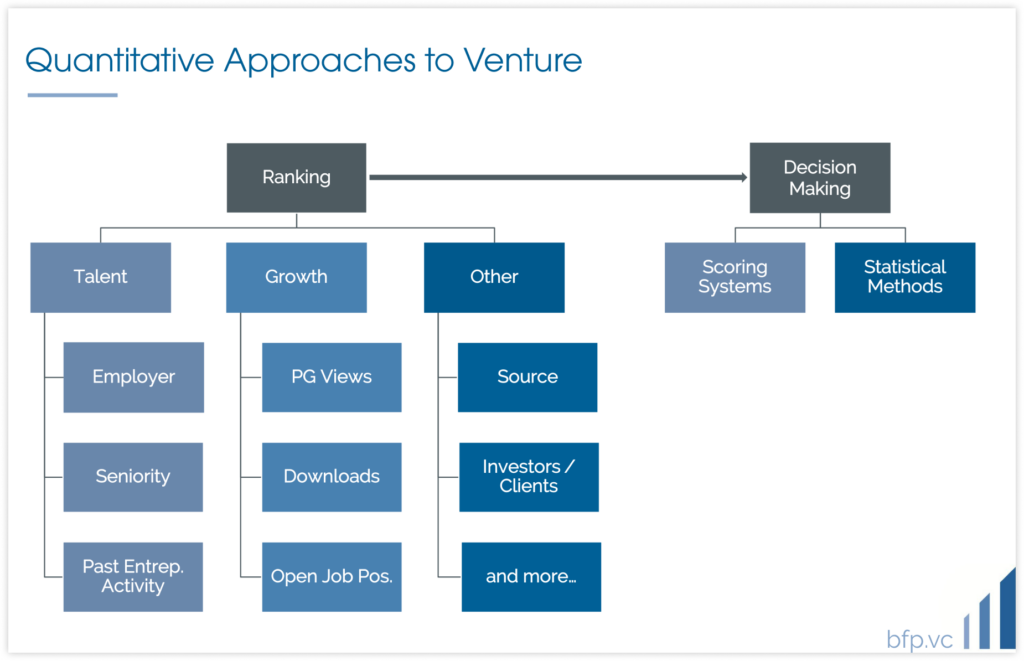
How Scouting and Filtering Models Work
Scouting and filtering models are used to insert into a system potential investments and to rank them. Data input are mainly related to three main aspects of a startup:
· Human Resources: The software scrapes data from a set of users in a platform and adds them to the dealflow according to a specific trigger event. The simplest example is a senior executive of a tech company leaving his/her position; when that happens, the software will tell the manager that such person could be starting something new- Most of the times the model will then rank talents based on two criteria:
§ Level or seniority
§ Previous activities and achievements (e.g. repeated founder, amount raised, exit value)
· Growth: Proxys can be found in different ways from several public sources. Examples are search analytics, page views, number of downloads, followers on social media, number of employees
· Other qualitative indicators such as previous investors, source or news coverage
Based on these input, the model then creates a ranking which is visualized by the manager.
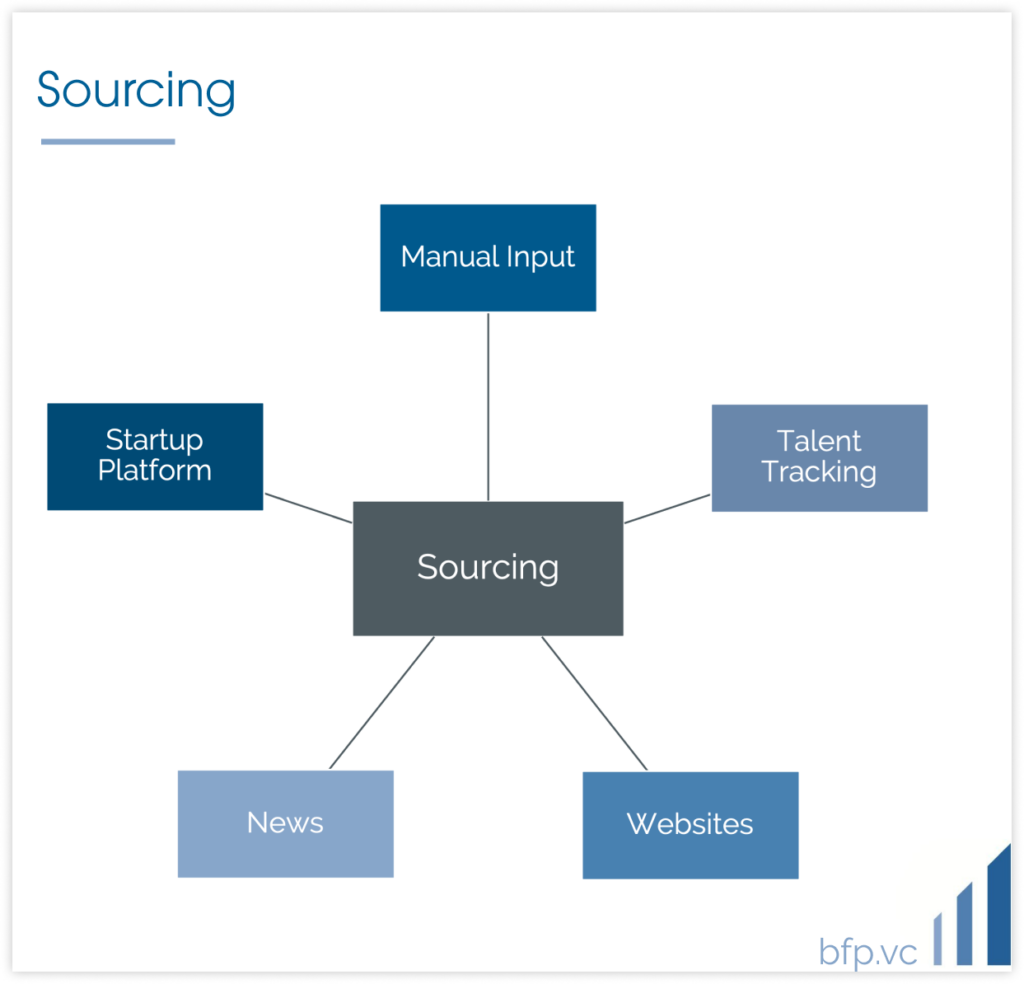
Limitations
There are three major interdependent problems with these approaches:
1. Building these models is not rocket science anymore and everyone can build them in a relatively short time. Basically, a larger firm with more management fees can build the same thing in a better way. If the determinant of a competitive advantage resides in the amount of money you can spend, there will always be somebody else who can spend more
2. In basically all the models we have seen so far, the output is actually somehow obvious. Is it useful an algorithm that tells a manager to chase successful repeated founders or senior executives from tech companies? Those are entrepreneurs chased by everyone anyway, with or without artificial intelligence
3. Venture is a game of innovation and outliers, which are by definition something different from the past. Future innovations do not necessarily follow the same path of previous ones, both in terms of type of products/service and of the people behind them. Patterns of success in venture capital are inherently dynamic, meaning that by the time a certain pattern leads to success it could already be obsolete
Our View
As a Limited Partner in early stage VC we definitely recommend managers to start experimenting with these tools but also to be extremely careful when relying to them.
Especially for emerging managers who are thinking about fundraising, our advice is to build a compelling narrative around your unique differentiation rather than around an AI model which everyone else can replicate.
Part 2 will discuss our view on quantitative approaches to investment decision making.
—
About Marco Cesare Solinas
Marco is an Analyst at Blue Future Partners, where he is responsible for sourcing and analysing new investment opportunities. He is passionate about Technology and Venture Capital and making an impact with investments. He focuses on both direct and indirect investments.
Previously, he has built an international and multicultural background across Italy, US, Germany, Turkey and Malaysia. Marco holds a CEMS Master’s in International Management and a Bachelor´s in Economics and Finance from Bocconi University.
Linkedin — Twitter — Medium